TL;DR
- Vector Databases and retrieval-augmented generation (RAG) are cornerstones for LLM-powered apps that rely on real-time knowledge retrieval.
- GPU Efficiency, batching, quantization, and parallelism, drastically cuts costs and boosts throughput.
- Pipeline Orchestration with frameworks like LangChain or LlamaIndex, combined with robust MLOps, prevents chaos as LLM applications grow in complexity.
- Continuous Monitoring of GPU usage, token consumption, and response quality is vital to avoid runaway spending and unexpected latency spikes.
- External Contract Developers can provide specialized skills—such as optimization, vector DB integrations, or deep GPU knowledge, without weighing down headcount.
The Production Gap in LLM Deployments
Deploying Large Language Models (LLMs) at scale poses unique engineering challenges. Demos or small proofs-of-concept rarely reveal the full complexity of running advanced AI in production, where cost, reliability, and performance must be optimized simultaneously. If you’re a CTO at a mid-sized company looking to roll out LLM features, you need an approach that balances all these factors while remaining flexible and maintainable over time.
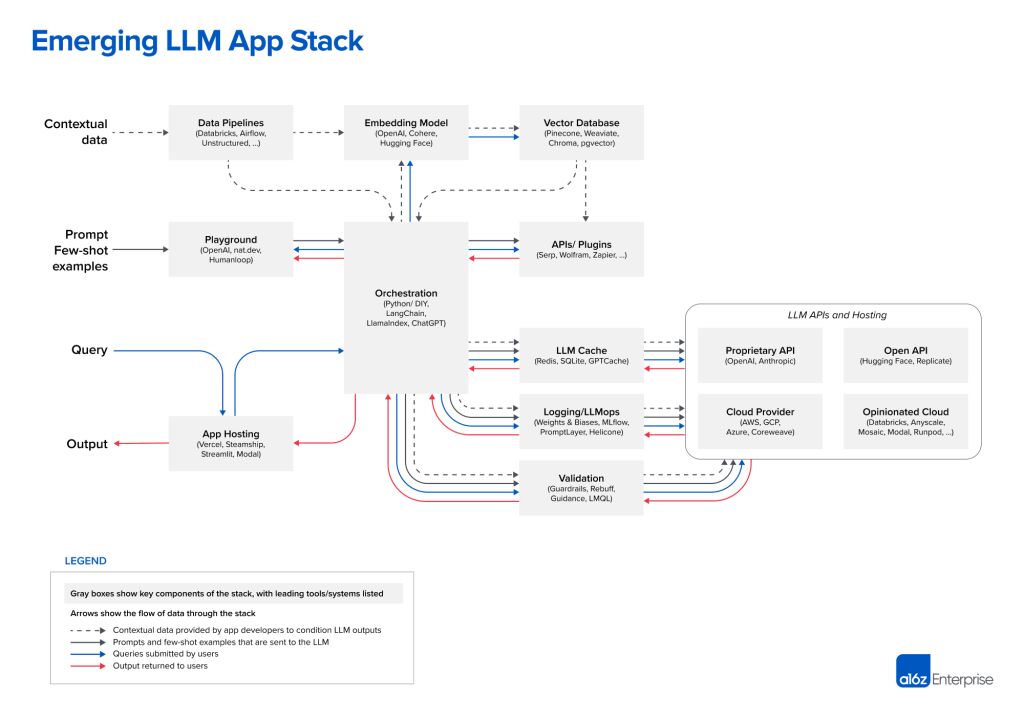
Core Infrastructure Challenges
1. Vector Search Integration
A growing number of LLM applications implement retrieval-augmented generation (RAG) to supply the model with domain-relevant context. This usually involves a vector database (e.g., Pinecone, Weaviate, Chroma, or Qdrant) where documents or data chunks are stored as embeddings for quick semantic lookup.
To scale this component effectively, ensure:
- Low-latency vector queries: Aim for sub-100ms retrieval, especially if your LLM workflow is synchronous.
- Robust indexing strategy: Keep embeddings updated as your data changes, possibly using Airflow or Kubernetes CronJobs.
- Integration with LLM orchestration frameworks: Tools like LangChain or LlamaIndex can abstract embedding creation, retrieval, and chunking, so you can focus on application logic.

2. GPU Utilization and Efficiency
Serving LLMs can be prohibitively expensive if GPUs are idle, overloaded, or suboptimally configured. Common tactics include:
- Batching: Combine multiple inference requests in one forward pass to boost GPU throughput. (Too large a batch can raise latency for each request, so tune carefully.)
- Quantization: Reducing weight precision from 16-bit to 8-bit or 4-bit can deliver 2–4× gains in inference speed and dramatically shrink memory usage.
- Auto-scaling: Dynamically allocate additional GPU pods or servers as traffic rises, then scale down during off-peak periods to cut costs.
- Parallelism: Employ tensor or pipeline parallelism if a single GPU can’t handle your largest models.
Below is a brief example of a Kubernetes HorizontalPodAutoscaler (HPA) configuration that reacts to GPU utilization. This is not an official API object in older K8s versions—some implementations rely on custom metrics or DaemonSets that feed GPU metrics to the HPA.
apiVersion: autoscaling/v2
kind: HorizontalPodAutoscaler
metadata:
name: llm-inference-hpa
spec:
minReplicas: 1
maxReplicas: 10
scaleTargetRef:
apiVersion: apps/v1
kind: Deployment
name: llm-inference-deployment
metrics:
- type: Pods
pods:
metric:
name: gpu_utilization
target:
type: AverageValue
averageValue: "70"
In the snippet above, gpu_utilization
is a placeholder metric that you would expose via a custom metrics pipeline (e.g., Prometheus with GPU exporters). When GPU utilization on average exceeds 70%, the HPA spins up new pods.
3. Orchestrating Complex LLM Pipelines
Production-grade LLM applications typically require multiple steps: data retrieval, user input parsing, calling the model, post-processing (including potential function calls), and logging. Frameworks such as LangChain or LlamaIndex manage these steps in a single code interface, while general-purpose workflow managers (Airflow, Prefect) or microservice architectures coordinate the bigger picture.
Crucial elements include:
- Multi-step inference logic: Tools like LangChain define “chains” or “agents” that handle retrieval, generation, and action-handling in one coherent pipeline.
- Scheduled workflows: Use Airflow or CronJobs to handle regular embedding updates, data ingestion, or nightly indexing.
- Microservices: Split your system into specialized services (embedding generation, inference, post-processing) so each can scale independently.
MLOps Essentials for LLM Deployment
Deployment with CI/CD and Canary Releases
Containerize your models for reproducibility and set up a continuous integration pipeline. When you push an updated model or a new inference logic version, it should pass automated tests (for accuracy, latency, etc.) before rolling into production. Canary releases let you gradually shift traffic to a new model, watching metrics such as latency, error rate, and user satisfaction in near real-time.
Observability and Prompt-Level Logging
Traditional server metrics remain important—CPU, GPU, memory, I/O—but LLMs also require monitoring of prompt-level data. Tracking inputs and outputs at scale helps spot anomalies like hallucinations or extreme token usage. Third-party solutions like WhyLabs, Gantry, or Neptune.ai can handle specialized LLM observability, but you can also build your own system on top of Prometheus and centralized logging (e.g., Elasticsearch or Loki).
Feedback Loops and Retraining
LLMs drift over time if the user domain or data distribution changes. Implement feedback loops by collecting user ratings or analyzing engagement metrics. Regularly incorporate new examples or corrected responses into your fine-tuning or distillation pipelines. This iterative cycle sustains performance and relevance, particularly in dynamic domains.
Governance and Compliance
For industries subject to data privacy laws or auditing requirements, log every prompt–response interaction and store relevant metadata in an immutable database. Implement checks for biases or inappropriate content, and maintain an internal process for prompt adjustments if the model outputs become problematic.
Real-World Examples: Key Lessons
- Meta discovered that once LLM-based features moved past demos, they needed hierarchical caching and dynamic scaling to manage highly variable loads.
- LinkedIn used model distillation to shrink the cost and latency of a skills-extraction feature, retaining nearly the same accuracy as the original large model.
- Mercari demonstrated the power of 8-bit quantization, cutting a GPT-scale model’s size by 95% and reducing inference cost by 14×.
- Dropbox reduced calls to the LLM with a multi-tier caching system that short-circuits repeated queries.
- Replit tackled cost challenges by accelerating container loading times for spot GPU instances, avoiding idle resource overhead.
Each story highlights how caching, batching, quantization, and advanced orchestration can transform an expensive or slow prototype into a production-ready service.
Consider Contract Developers for Specialized Expertise
Despite the best efforts of internal teams, scaling LLMs may demand niche expertise—like GPU scheduling, distributed vector indexing, or advanced MLOps. Contract developers or freelance engineers experienced in precisely these areas can speed up deployments without burdening your hiring pipeline. To integrate them effectively:
- Clearly scope deliverables (e.g., “reduce latency by 30%,” “migrate embeddings to a new vector DB”).
- Provide access to necessary documentation, domain knowledge, and Slack channels for real-time collaboration.
- Emphasize thorough handoff and code documentation to prevent knowledge silos once the contract ends.
Action Steps: Prioritizing Your Next Moves
- Audit Current Bottlenecks
- Profile GPU usage, measure end-to-end latency, and track token consumption to locate waste.
- Implement Quantization or Distillation
- If you’re using a large generic model, try compressing or switching to a more specialized, lightweight variant.
- Adopt a Vector Database
- For any retrieval-augmented pipeline, move beyond ad-hoc embedding queries to a robust vector search store.
- Set Up Observability Dashboards
- Integrate GPU metrics, model performance, and cost data into a single pane of glass.
- Explore Specialized Help
- Identify holes in your team’s expertise—whether that’s GPU ops, MLOps frameworks, or vector indexing—and consider contracting experts to fill the gap.
Conclusion: Shaping an LLM Strategy That Lasts
Scaling LLMs is both an engineering and operational challenge. You need to build pipelines that harness advanced AI features while diligently managing costs, maintaining high uptime, and delivering the performance your users expect. Balancing these competing factors requires technical depth in GPU utilization, data pipelines, and MLOps—along with a willingness to iterate and experiment.
By integrating best practices such as batching, caching, quantization, and microservice orchestration, you can push an LLM deployment from a successful prototype to a stable, production-grade system. Contract developers can accelerate the process if your internal team lacks certain specialized skills. The payoff is a well-architected AI service that differentiates your business while staying cost-effective, reliable, and poised for future evolution.
If you’re ready to optimize or expand your LLM deployment, request engineer profiles to find seasoned pros who can address your most pressing AI challenges.
The post Scaling AI Infrastructure for LLMs: Best Practices for Mid-Sized Companies appeared first on Gun.io.